May Wang, CTO IoT Security, Palo Alto Networks
“AI’s Impact in Cybersecurity” is a blog series based on interviews with experts at Palo Alto Networks and Unit 42 who have roles in AI research, product management, consulting, engineering, and more.
In our newest blog, we interview May Wang, CTO IoT Security, Palo Alto Networks, for her take on how AI is impacting the field. We also explore a short retrospective of how “The Internet of Things” (IoT) got its name, and take a brief look at current IoT usage data.
How Did We Get Here?
According to internet lore, in 1982 (or thereabout), four students from Carnegie Mellon University's School of Computer Science developed the world's first “network-connected” appliance. And because necessity is the mother of invention, those resourceful fellows didn't program the “Next Big Thing,” but rather a campus Coke® machine to remotely check soda inventory from their desks in order to determine when to restock the carbonated canisters.
Moving ahead to 1999, Kevin Ashton is cited to have first coined the phrase, “Internet of Things" as the title of his presentation, while working as a brand manager for Procter & Gamble (P&G)…because lipstick. Noting a discrepancy in inventory reports for a new line of Oil of Olay® cosmetics, he became determined to rectify current barcode scanning systems with a more holistic and realistic view of P&G’s supply chain.
Fueled by his vision of wireless smart packaging, he would go on to partner with MIT's Media Lab and Auto-ID Center to study nascent RFID capabilities. He explained:
“Today's computers, and therefore the internet, are almost wholly dependent on human beings for information. Nearly all of the roughly 50 petabytes of data available on the internet were first captured and created by human beings by typing, pressing a record button, taking a digital picture, or scanning a barcode. Conventional diagrams of the internet include servers and routers and so on, but they leave out the most numerous and important routers of all – people. The problem is people have limited time, attention and accuracy. All of which means they're not very good at capturing data about things in the real world.”
Data, Data Everywhere
Fast forward to 2024, where, with the ability of current AI capabilities, we are able to realize the potential of ingesting and analyzing vast amounts of data (from multiple sources) into security tools and platforms.
At Palo Alto Networks, our own security operations center ingests 100TB of data per day from endpoint, network and cloud logs, including third-party apps (e.g., Okta, Google Workspace, Workday, GCP, AWS, etc.) Using AI and machine learning in our own products, such as Cortex XSIAM, enables our analysts to correlate and filter alerts, as well as make decisions about which alerts are critical enough to require further investigation.
Protecting our customers is always a top priority, and as the IoT landscape continues to evolve, staying informed about the latest trends and challenges is crucial. This brings us to an important point highlighted in the 2023 Benchmark Report on IoT Security:
“Recent studies estimate that enterprises today have an average of more than 3,000 connected IoT devices, up from just under 700 in 2020. This rapid increase is being driven by a number of factors, including the falling cost of sensors and the proliferation of low-power connectivity options, such as Bluetooth Low Energy and LoRa. Recent studies also predict that, not surprisingly, the number of connected IoT devices will continue to rise in the coming years, reaching an average of over 9,000 per enterprise by 2025. However, this growth will not be uniform across all industries, with manufacturing and healthcare expected to see the largest increases in connected devices.”
As IoT continues to expand, with an average of 22 smart devices per household, according to Deloitte’s Connectivity and Mobile Trends (CMT) survey, the challenges of securing these devices have become increasingly complex.
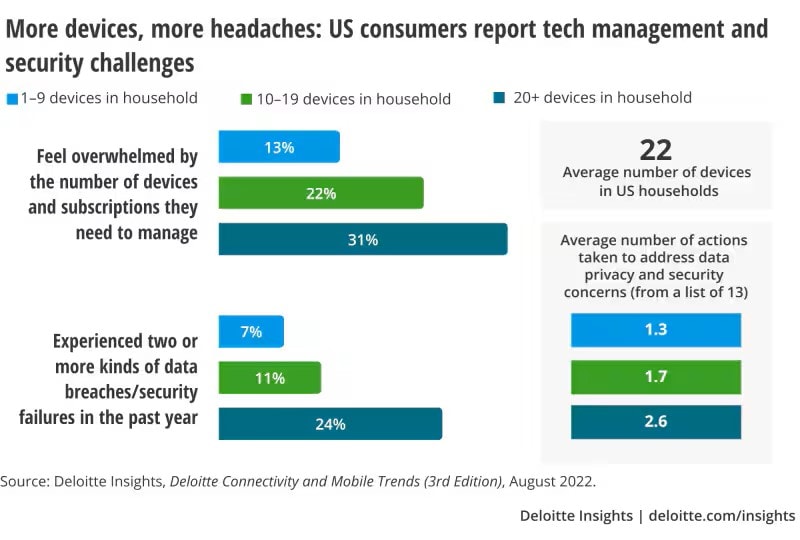
Leveraging AI to Overcome IoT Security Challenges
In our interview, Wang emphasized the unique security challenges posed by IoT devices, stating, "There are tons of challenges for these IoT devices compared to traditional IT devices." These challenges include the large quantity and variety of devices, large attack surfaces, long shelf times and lack of self-protection capabilities.
One of the most significant challenges is the sheer quantity and variety of IoT devices. With the rapid proliferation of connected devices, organizations are facing an ever-growing number of devices to manage and secure. Unlike traditional IT devices, which are typically designed with security in mind, many IoT devices lack basic security features, such as encryption, and are often deployed with default settings and weak passwords. This makes them vulnerable to various types of attacks, such as unauthorized access, data theft and botnet recruitment. Additionally, IoT devices are often connected to networks that are accessible from the internet, further expanding their attack surface.
Another challenge is the long shelf life of IoT devices. Unlike smartphones and laptops, which are frequently updated and replaced, IoT devices often remain in use for several years without regular security patches or updates. This leaves them exposed to newly discovered vulnerabilities and threats. As a result, organizations are faced with the daunting task of securing devices that may have outdated software and hardware.
Furthermore, many IoT devices lack self-protection capabilities. They often have limited processing power, memory and storage, making it difficult to implement robust security measures directly on the devices. Traditional security solutions, such as antivirus software and firewalls, may not be suitable for resource-constrained IoT devices. This lack of built-in security mechanisms leaves IoT devices reliant on network-level security controls, which can be challenging to implement and manage effectively.
Due to these challenges, Wang sees AI as a powerful tool to address these obstacles:
"AI is a perfect match for ingesting IoT data, as the devices generate such huge amounts of data that we couldn't access before, or we couldn't access in real time. IoT provides huge amounts of data and feeds it into AI. It's very hard for a human being to really scale up a solution to these billions of IoT devices, but AI is really, really good at it."
AI-Powered Device Discovery and Anomaly Detection
At Palo Alto Networks, AI is being leveraged for two primary purposes in IoT security – device discovery and anomaly detection. By using AI to automatically identify and catalog IoT devices, security teams can gain real-time visibility into their environment, a task that was previously manual and very time-consuming. Additionally, AI models can be trained on the vast amounts of data generated by IoT devices to detect anomalies and potential threats.
Wang also addressed the challenges of securing the AI supply chain and preventing attacks, such as data poisoning. Data poisoning is a type of attack that occurs during the training phase of machine learning models. In this attack, an attacker manipulates the training data by injecting carefully crafted samples or by modifying existing data points with the goal of compromising the model's performance or causing it to behave in a way that benefits the attacker.
Wang went on to highlight the importance of designing AI models with security in mind, protecting training data, and being aware of new threats, such as prompt injection in the era of generative AI.
When asked about best practices for integrating AI into existing IoT security architectures and workflows, Wang emphasized the importance of collecting the right data, building efficient infrastructure, and applying AI to the most effective use cases:
"Not only do we need to work on the models, we need to get the most efficient model, optimized model, best trained model… we need to get the right models, but we also need to work on multiple other factors, for example, data. We need to make sure we collect the right data, process the data correctly, bring in relevant data, and have the highest-quality data, et cetera.”
As the adoption of IoT devices continues to grow, Wang believes that AI will play an increasingly critical role in securing these devices. She predicted:
"Moving forward, I do see there is going to be further integration and leverage of AI for IoT. More scalable, more automatic, more intelligent and faster identification and detection. I think we’re going to see more benefits of AI for IoT security. Both platformization and Precision AI™ are particularly important for IoT security. IoT needs the platform to access different resources, and at the same time fuel into the platform with device data. When an IoT device is hacked, not only can we lose sensitive information, but also it can impact the operation, which can mean life or death in the cases of healthcare and critical infrastructure. Therefore, the accuracy of AI is extremely crucial.”
For cybersecurity professionals, staying ahead of the curve in understanding and implementing AI-powered solutions for IoT security will be essential. As Wang stated, "I think all we need to do is to face new challenges and figure out new solutions. And that's also where the opportunities will come." By embracing AI and working to address the challenges it poses, security teams can harness its power to protect the ever-expanding world of IoT devices.
What’s Next? Securing the Future with Precision AI
Recently, Palo Alto Networks announced Precision AI, a proprietary AI system that helps security teams trust AI outcomes by using rich data and security-specific models to automate detection, prevention and remediation with industry-leading accuracy. It incorporates the best of our AI capabilities:
- Machine Learning – Built into many of our products for more than a decade, ML allows our security applications to become more accurate at preventing, predicting and remediating security problems by using precise, defined historical and current data as input to predict novel situations.
- Deep Learning – Helps us build predictive models to anticipate and detect security issues in real-time by learning from massive amounts of security data.
- Generative AI – We use GenAI to enable our tools to “speak human,” simplifying UX and summarizing large volumes of threat intelligence. We do this through our co-pilots, built on our own highly controlled datasets, which reduces mean time to resolution (“MTTR”).